10.2 Supplementing data with analysis#
10.2.1 Introduction#
A fact is something consistent with objective reality, or that can be proven with evidence. Dictionaries define a fact as something that can be shown to be true, exist, or have happened. Therefore, the usual test for a statement of fact is verifiability, whether it can be demonstrated to correspond to experience. Given that official statistics are the products of processes that incorporate objective, scientific methods, they can be used as a basis for fact verification.
Even though official statistics bring awareness to particular issues and are a basis for fact verification, it must be noted that facts, for the most part, are still subject to interpretation. A user of statistical data analyses them and interprets their meaning within his/her own particular context. It is generally agreed that an NSO should comment on its most important statistical outputs and use its detailed knowledge based on access to the microdata to comment on impressions created by them, particularly if those impressions are liable to be wrong. However, analyses provided by an NSO should avoid policy and political interferences and comments. In General, an NSO would restrict itself to comment on statistical correlations between data sets and not interfere with non-statistical causality analyses. Analysis performed by the NSO should be an integral part of the production process, along with quality management. However, in the end, only a fraction of this analysis will supplement the dissemination of the results of official statistics. Disseminated analysis results should refer to ways of looking at and talking about data without imposing definitive conclusions about what the data mean. This includes providing interesting breakdowns, contextual information, explanatory notes and commentaries to accompany disseminated data, thereby giving additional value to users.
Walter J. Rademacher β The future role of official statistics (π).
10.2.2 Analytical functions and outputs#
Analytical results underscore the usefulness of statistical outputs by shedding light on relevant issues. Statistical outputs often depend on analytical output as a major component because, for confidentiality reasons, it is not possible to release the underlying microdata to the public. Data analysis also plays a key role in data quality assessment by pointing to data quality problems. Analysis can thus be a trigger for future improvements in the statistical production process.
Data analysis involves summarising the data and ensuring that it provides clear answers to the questions that initiated the statistical process. Often, it consists of analysing tables and calculating summary measures, such as frequency distributions, percentages, means and ranges. For sample surveys, it includes a description of the observed units, a selection of statistical outputs (tables, charts, measures of spread, models, etc.), and/or a description of the population and tests of hypotheses about it, in which case the sample design must be properly accounted for.
In addition to analysing the statistical data from a user perspective, NSOs should also continuously monitor and analyse the underlying statistical processes to ensure their stability and enable continuous improvement of their quality, as was discussed in Chapter 7.2 β Measuring and analysing user satisfaction and needs. Analysis may be undertaken at various stages in the statistical process, and by various organizational units, depending upon the overall organizational structure of the NSO, as further discussed in the following sections.
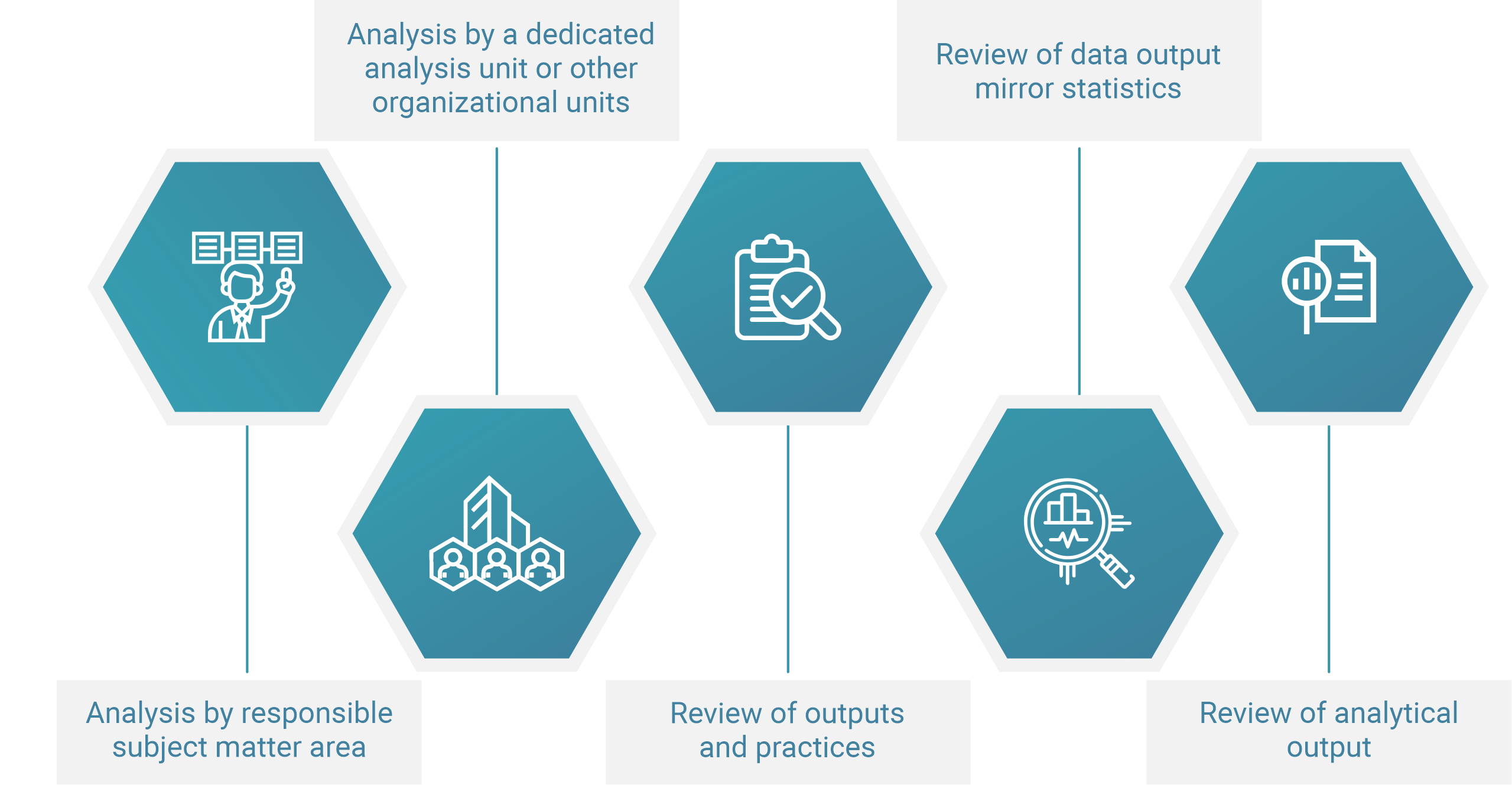
Analysis by responsible subject matter area
The subject matter specialist may perform analysis during the processing of statistical data and prepare the statistics for dissemination. The analysis may begin with exploratory data analysis and macro-editing methods described in Chapter 9.2.6 β Processing survey. This form of analysis, which may be termed preliminary analysis, involves summarising the raw data and investigating any data discrepancies. All preliminary outputs should be analysed in this way, to determine data consistency and to direct further analysis.
The preliminary analysis includes macro editing, drill-downs to unit data, tabulation, exception reporting and assessment of results against results from previous periods and related data sources.
During this phase, subject matter experts also perform the additional analysis required to complete the quality reports. This type of analysis provides a better understanding of the properties of a dataset and the underlying phenomena. It also helps identify potential errors in the processing, helps improve weights for sample surveys and identifies areas for further improvement, for example, parts of a questionnaire that may benefit from wording improvements.
The key elements of analysis are performed just before dissemination, while the data are being tabulated and prepared for release. The analysis may include preparing a summary of key findings of the release, preparing the explanatory notes that provide detailed information about properties of the dataset, and preparation of charts to accompany the release. The analysis should try to mimic the users of data, and, by replicating the procedures that they may use, try to further check the consistency of released figures. Commonly, comparisons are made with the results with previously released statistics and with other data sources. Every effort should be made to reflect usersβ interests and perform additional checks of data that may be of particular interest to them.
Even though these types of analysis are usually done by the subject matter specialist, there are NSOs where tabulation is done by the IT department and charts and commentaries are produced by the dissemination department. For sample surveys, some analyses such as a non-response analysis (see Chapter 9.2.7 β Respondent relations and communications for more details) may be performed either by the subject matter area or the organizational unit responsible for processing, or other specialised units, such as methodology. Subject matter experts may also perform time series analysis and analysis for confidentiality and disclosure control (as described later in this chapter). These types of analysis may also be performed by methodology experts or jointly with them.
Analysis by a dedicated analysis unit or other organizational units
This section describes options for organizing the expert knowledge (such as sampling techniques, analysis methods, IT and dissemination options) required for statistical analysis within an NSO. As this knowledge is needed across the full range of statistical processes, staff with such knowledge are usually located in a dedicated analysis unit, particularly in larger and well developed NSOs. In many smaller NSOs, this may not be possible.
Depending on the type of the statistical process, additional analyses may also be performed by other experts in specialised units. For example, an expert in the statistical business register unit may analyse the classifications assigned to reporting units and perform coverage tests in order to determine the representativeness of responses within subgroups of the population as discussed in Chapter 12.4 β Frames for informal sector surveys. A sample design expert may analyse weights to perform nonresponse adjustments and improve estimates for sample surveys, as discussed in Chapter 9.2 β Sample surveys and censuses. Experts in an analysis unit may perform time series analysis and seasonal adjustment. An IT or data management expert may apply disclosure control measures discussed in Chapter 12.8.5 β Confidentiality and disclosure control. Some larger offices may also have a dedicated experimental statistics unit that may deal with innovative sources and Big Data issues. This unit often requires different skillsets, combining statistical knowledge with extensive technological skills, as discussed in Chapter 9.2.9 β Survey staff training and expertise.
Regardless of the statistical process, further analysis of the coherence and consistency of the statistical output is performed by the unit that specialises in the corresponding analytical framework (if there is one). The most notable example is the national accounts unit, where data from a wide range of sources are brought together and analysed side by side as further discussed in Chapter 10.4 β National accounts).
Eurostat, Handbook on improving quality by analysis of process variables (π).
Review of outputs and practices
In their quest for continuous improvement, an NSO should be open to a broad review of its outputs and evaluate its practices. An internal review of a publication before release is a common practice. It engages the senior officials of the organization and fosters cross-subject review and criticism/critiquing. For example, a release of statistics on employment and unemployment may be effectively reviewed by those responsible for industry and trade statistics or by national accountants. This type of review is usually performed regularly. It is particularly important if statistics being released tend to attract a significant number of questions (or frequently repeating questions) after the release.
A more formal process should be reserved for larger analysis efforts, such as analyses associated with a new survey of family incomes and expenditure, a new economic census, or a new population census. In addition to engaging multiple experts and units internally, it is beneficial to persuade members of the academic community to take part in a review process. The goal of the review is to judge whether the statements made are fully supported by evidence; whether the most important inferences based on the new data available have been taken into account; and whether the methods used stand up to close scrutiny in the face of current knowledge. Additionally, publications are also scrutinized as part of periodic external reviews that may result in recommendations that can vastly improve the quality of future statistical outputs. In all cases, such reviews are likely to involve significant analysis of statistical output and metadata. Such reviews may be performed as national, regional and/or international exercises. They may focus on the entire NSS, or a particular subject matter area. Regional or international reviews usually check the application of international standards, such as the Global review of the implementation of the Fundamental principles of official statistics (π). The scope of a national review may vary according to the structure of the NSS. In a centralised system, a review may check the quality of outputs, while in a decentralised system, it may check both the quality of outputs and the application of principles. (More details are provided in Chapter 8 - Quality Management).
Review of data output β mirror statistics
Mirror statistics refer to the situation where flow statistics between two countries are compared. For example, the exports of country A to country B (measured by county A) are compared with the imports of country B from country A (measured by country B). The aim is to detect causes of bilateral asymmetries. Apart from detecting asymmetries, the mirror statistics methodology may be used to derive estimates or impute a missing variable of flow statistics for a country using data from the partner country. Mirror statistics are commonly used for foreign trade statistics, and migration statistics, where the mirror data flows from partner countries can be used to assess the quality of the data and eventually compile estimates for the country.
Even though mirror statistics are useful in filling data gaps, their prime use should be for review purposes. The most common process of mirror statistics review is performed when NSOs of two or more countries agree to compare the results and the underlying microdata to improve the quality, for example through subsequent improvements in registration and classification procedures.
UNECE - Statistics on International Migration: A Practical Guide for Countries of Eastern Europe and Central Asia (π);
UNESCAP - Asymmetries in International Merchandise Trade Statistics: A case study of selected countries in Asia-Pacific (π).
Review of analytical output
Review of analytical output is usually performed by external reviewers or through a peer-review process. It consists of checking the processes and procedures that accompany the statistical releases. GSBPM provides a framework that can be used to systematically review the stages of production. Statistical areas are usually reviewed using an audit-like approach, as each step should be well documented to enable the replication of results. There are also examples where reviews on statistical processes and products are conducted by function such as dissemination, sampling or the use of statistical registers, and not by statistical domain. Further, systematic reviews of whole statistical systems are occasionally commissioned to assess the effectiveness of the statistical system and its governance models.