9.5 Big Data#
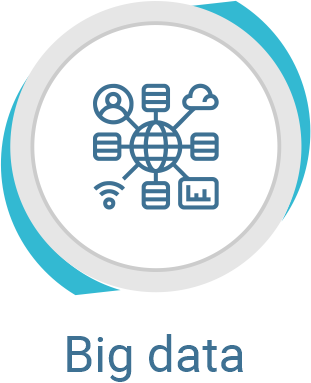
The term Big Data refers in general to data generated by business transactions, social media, phone logs, communication devices, web scraping, sensors etc. (see Chapter 15.2.7 — Big Data). For a general introduction to the notion of Big Data, see the book Big Data by Viktor Mayer-Schönberger and Kenneth Cukier (2013).
Big Data has attracted considerable and increasing interest from NSOs regarding the potential to complement traditional statistics. This is particularly important in the context of the need to measure and monitor progress towards the Sustainable Development Goals (SDGs) and other targets.
Big Data has the potential to supplement, complement or partially replace, existing statistical sources such as surveys, or provide complementary statistical information but from other perspectives. It could also improve estimates or generate completely new statistical information in a given statistical domain or across domains.
Big Data is widely used in the commercial sector for business analytics, but there is less evidence of its use thus far in the world of official statistics. Despite the high expectations for using Big Data, the reality is that while the technology needed to process these huge data sets is available and maturing, the biggest obstacle for an NSO is often to gain access to the data. This lack of access can be due to reluctance of a business to release their data, legal obstacles, costs or concerns about privacy. However, where Big Data is accessible for an NSO, such as websites or sensors administered by public administrations, such as road sensors, it is already successfully used for experimental or even official statistics.
Box 6: Big Data and Data Science - new ways of working in official statistics
At about the time that the UN Secretary-General Ban Ki-Moon released the Data Revolution report (🔗) in 2014, the UN Statistical Commission decided that the statistical community needed to explore in more detail the benefits and challenges of using Big Data for official statistics. The private sector was moving fast in exploiting large amounts of data from digital devices for market analysis and improving business operations, and the statistical community should not stay behind.
In March 2014, the Committee of Experts on Big Data and Data Science for Official Statistics (🔗) was created. It distributed its work over about a dozen task teams (🔗) looking into the use of earth observations, mobile phone data, scanner data, social media data and AIS vessel tracking data, as well as cross-cutting issues regarding data access, privacy-preserving techniques, IT infrastructure, communication and awareness-raising, and capacity development. Since its inception, the Committee released guidelines on all the mentioned areas, developed training materials and conducted numerous seminars and workshops.
The achievements of the Committee were not only reported to the Statistical Commission (🔗) but also exhibited at annual Big Data Conferences in Beijing (2014), Abu Dhabi (2015), Dublin (2016), Bogota (2017) and Kigali (2019). Due to the Covid-19 pandemic, the planned conference in Seoul (2020) was conducted as a virtual event.
In Bogota (November 2017) (🔗), the Committee decided that a Cloud-based collaborative environment should be developed for the statistical community to conduct joint Big Data projects and to share data, methods, applications and training materials. The Office for National Statistics of the United Kingdom took the lead and built the UN Global Platform (🔗) in the period 2018-2020, then handed it over to the UN community in June 2020. National statistical institutes could now use satellite data or AIS vessel tracking data with corresponding Cloud, method, and developer’s services to run big data projects. However, necessary skills in data engineering and data science and the use of various new data sources were lacking. Therefore, four Regional Hubs for Big Data (🔗) in support of the UN Global Platform were established in Brazil (Rio de Janeiro), China (Hangzhou), Rwanda (Kigali) and UAE (Dubai). Through these hubs capacity building activities were brought to all world areas.
The Expo2020 event in January 2022 in Dubai (🔗) not only showcased the ceremonial launch of the Regional Hub for Big Data in the UAE, but also highlighted the other regional hubs as well as the strong commitment of the hubs to collaborate with each other for the benefit of building capacity in the use of Big Data and Data Science in all countries. Some reflections on new ways of working in official statistics can also be found in a December 2021 article (🔗) by the UN Statistics Division in the Harvard Data Science Review.
9.5.1 Types of Big Data#
There are several categories of Big Data.
Structured data
All the data received from sensors, weblogs and financial systems are classified under machine-generated data. These include medical devices, GPS data, data of usage statistics captured by servers and applications and the huge amount of data that usually move through trading platforms, to name a few. Human-generated structured data mainly includes all human input data into a computer, such as his name and other personal details. When a person clicks a link on the internet, or even makes a move in a game, data is created.
Unstructured data
While structured data resides in the traditional row-column databases, unstructured data is the opposite- they have no clear format in storage. The rest of the data created, about 80% of the total, account for unstructured Big Data. Until recently, there was not much to do to it except storing it or analysing it manually. Unstructured data is also classified based on its source, into machine-generated or human-generated. Machine-generated data accounts for all the satellite images, the scientific data from various experiments and radar data captured by various facets of technology. Human-generated unstructured data includes social media data, mobile data and website content. This means that the pictures we upload to Facebook or Instagram the videos we watch on YouTube and even the text messages we send contribute to the mass of unstructured data.
Semi-structured data
Information that is not in the traditional database format as structured data but contains some organizational properties that make it easier to process is included in semi-structured data. For example, NoSQL documents are considered semi-structured since they contain keywords that can be used to process the document easily.
9.5.2 Big Data sources
There are broadly four sources of Big Data.
Transactional data is generated from all the daily transactions that take place both online and offline. Invoices, payment orders, storage records, delivery receipts – all are characterized as transactional data. However, data alone is almost meaningless, and most organizations struggle to make sense of the data they are generating and how it can be put to good use. Business transactions: Data produced from business activities can be recorded in structured or unstructured databases. With a big volume of information and the periodicity of its production (because sometimes this data is produced at a very fast pace), thousands of records can be produced in a second when big companies like supermarket chains are recording their sales.
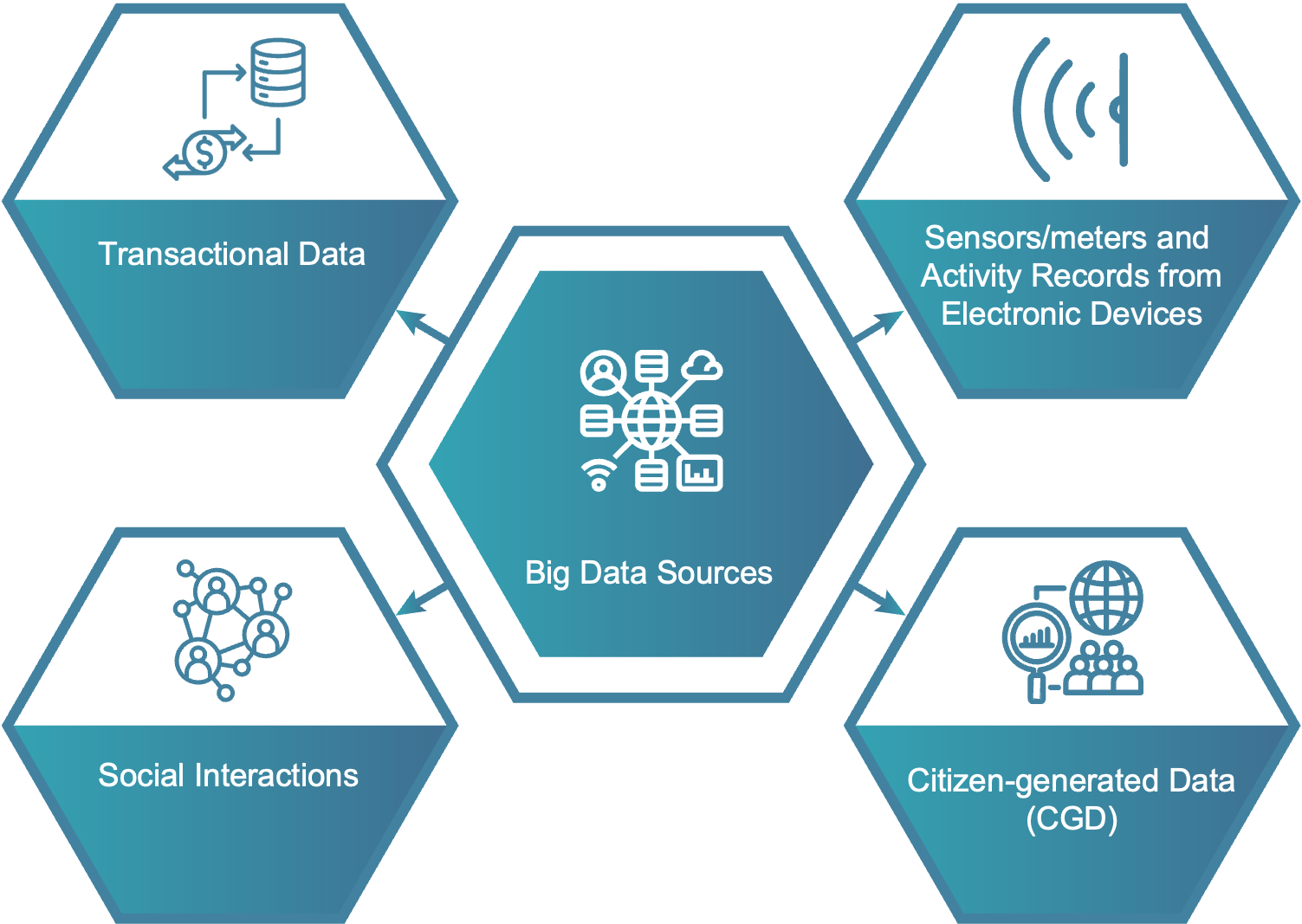
Sensors/meters and activity records from electronic devices: the quality of this kind of source depends mostly on the sensor’s capacity to take accurate measurements in the way it is expected. Machine data is defined as information generated by industrial equipment, sensors installed in machinery, and even web logs that track user behaviour. This type of data is expected to grow exponentially as the Internet of Things (IoT) grows ever more pervasive and expands around the world. Sensors such as medical devices, smart meters, road cameras, satellites, games, and the rapidly growing Internet of Things will deliver high velocity, value, volume, and data variety shortly.
Social interactions: this covers data produced by human interactions through a network. The most common is the data produced in social networks. This kind of data relies on the accuracy of the algorithms applied to extract the meaning of the contents commonly found as unstructured text written in natural language. Some examples of analysis made from this data are sentiment analysis, trend topics analysis, etc. Social data comes from the Likes, Tweets & Retweets, Comments, Video Uploads, and general media uploaded and shared via the world’s favourite social media platforms. This data provides invaluable insights into consumer behaviour and sentiment and can be enormously influential in marketing analytics. The public web is another good source of social data, and tools like Google Trends can be used to improve the volume of Big Data.
Citizen-generated data (CGD) is data produced by non-state actors under the active consent and participation of citizens to primarily monitor, demand or drive change on issues that affect them directly. CGD can be an innovative data source (secondary data source) for the production of official statistics and be leveraged to support the effective tracking of progress on the Sustainable Development Goals (SDGs).
UNECE has developed a multi-layer classification of Big Data sources (🔗) with 24 categories at the lowest level.
9.5.3 Challenges accessing and processing Big Data
Accessing Big Data
According to the GLOS guidance. Article 6.1 ‘Producers of official statistics shall be entitled to access and collect data from all public and private data sources free of charge, including identifiers, at the level of detail necessary for statistical purposes. In the longer term, the goal is to regulate this access in the statistical law.
There are several potential barriers an NSO has to overcome in gaining access to Big Data sources. These include the following:
concerns on the part of private companies about losing their competitive advantage;
legal constraints concerning privacy and confidentiality of client information;
businesses have recognised the value of their data and are not prepared to just give it away;
the costs of setting up the necessary infrastructure and training staff for a non-core business-related activity.
NSOs need to overcome such legal requirements and seek agreement with businesses to gain access to Big Data for statistical purposes. If agreements can be struck with the businesses that own the data, several business models can enable data exchange between private corporations and an NSO. The PARIS21 paper “Access to New Data Sources for Statistics: Business Models and Incentives for the Corporate Sector” (🔗) lists the following models:
In-house production of statistics: the in-house production of statistics model is, in many ways, the most conventional or standard model. It is used by most NSOs today and, as such, comes with a known set of risks and opportunities. On the positive side, the model allows the data owner to maintain total control over the generation and use of its raw data. User privacy can be protected through deidentification, and generated indicators can be aggregated sufficiently to be considered safe for sharing. From a safety or security point of view, the in-house production of statistics is the preferable option.
Transfer of data sets to end users: in this model, data sets are moved directly from the data owner to the end-user. The model gives the end-user significantly more flexibility on how the data is used. In general, raw data is de-identified, sampled and sometimes aggregated to avoid possible re-identification. Efforts to de-identify need to ensure that data cannot be re-identified by crossing it with external data. Because de-identification is never absolute, even when the most sophisticated anonymizing techniques have been deployed, data in this model is generally released to a limited number of end-users, under strict non-disclosure and data usage agreements ensure a level of control and privacy.
Enabling remote access to data: in the remote access model, data owners provide full data access to end-users while maintaining strict control on what information is extracted from databases and data sets. In this model, personal identifications are anonymized, but no coarsening is made on the data. The data does not leave the premises of the data owner; rather, the end-user is granted secured access to analyse the data and compute the relevant metrics. The end-user is then permitted to extract only the final aggregated metrics once the data analysis has been completed. This method is often used in research, in specific partnerships between the data owner and a group of researchers, under very strict non-disclosure and data usage agreements. Strict monitoring of the input and output traffic on data storage devices is carried out to ensure no data is removed. The main incentive in this type of model is that users benefit from free research resources on their data.
Using trusted 3rd parties (T3Ps): in the Trusted 3rd party (T3P) model, neither the data owner nor the data user supports the security burden of hosting the data themselves. Instead, both parties rely on a trusted third party to host the data and secure access to the data source. The data is anonymized in the sense that hashing techniques protect personal identifiers. In addition, the end-user does not have direct access to the raw data. Instead, end-users must make a request for reports or other intermediate results to the T3P, which ensures protection of the data.
Moving algorithms rather than data. In this model, shared algorithms allow the reuse of software by several private data owners wishing to perform similar analytical functions on one or several data sets. For example, such a model may be effective when several national telecoms operators wish to estimate population density (or other population patterns) based on their collective data. The data sets from different operators do not need to be necessarily merged. Instead, while the analytical functions performed on each data set may be identical, the data sets themselves can remain separate and under separate control. Results can be arrived at independently by each operator, and the aggregated results can later be merged to arrive at a comprehensive national or regional analysis.
To gain reliable and sustainable access to Big Data sources, NSOs need to form strategic alliances with the data producers, which can be a lengthy process with no guarantee of success. Private corporations are aware of the value of their data, are unwilling to expend resources on activities that are not mission-critical, and that carry potential risks of business information and confidentiality breaches. Governments must enact legislation obliging corporations to make their data available to NSOs to use for the public good. However, this can take many years to put in place.
The issue of gaining access to privately held Big Data sources needs to be addressed at a supranational level, particularly as many of the companies generating Big Data are multinational concerns. The legal aspects are complex and difficult to solve legally at the country level. Moreover, NSOs are not the only organizations interested in gaining access to Big Data for public purposes. In the EU, for instance, a broad expert group (🔗) is looking into this issue. The EU has also provided some guidance (🔗), specifically mentioning statistics.
Challenges in processing Big Data
Data privacy
With Big Data, the biggest risk concerns data privacy. Enterprises worldwide make use of sensitive data, personal customer information and strategic documents. A security incident can not only affect critical data and bring a downward reputational effect; it can also lead to legal actions and financial penalties. Taking measures for data privacy is vital - as recent high-profile cases have shown, if not sufficiently protected this information can be used to profile individuals and be passed on to third parties leading to loss of consumers’ trust. Thus, NSOs need to ensure that data sources and indicators used are obtained without violating privacy or confidentiality regimes.
Costs
NSOs must also invest in security layers and adapt traditional information technology techniques such as cryptography, anonymisation, and user access control to Big Data characteristics. Even though that the access to data for NSO should ideally be free of charge as with access to administrative data, it may be necessary to pay the one-off cost of preparing the data transfer system such as an API.
Data quality
Big Data is often largely unstructured, meaning that such data sources have no pre-defined data model and does not fit well into conventional relational databases. Diverse structures also cause data integration problems as the data needed for analysis comes from diverse sources in various formats such as logs, call-centres, web scrapes and social media. Data formats will differ, and matching them can be problematic. Unreliable data: Big Data is not always created using rigorous validation methods which can adversely affect quality. Not only can it be inaccurate and contain wrong information but can also contain duplications and other contradictions.
Methods
Using Big Data may require new methods and techniques. For instance, new modelling techniques may be called for, especially if Big Data is used for producing early indicators or even nowcasting. Artificial Intelligence and deep learning techniques may be used for processing unstructured text messages or satellite images.
Data impermanence
An NSO cannot guarantee that a data source will be reliable as it has no control over or relationship with the data owner as with traditional data sources. Formats can change at any time without warning that can render data capture and subsequent processes that have been put in place by the NSO unworkable. Data sources can even disappear completely if the business rules generating the data are changed.
Data gaps
The SDGs have a fundamental commitment to leave no-one behind. Vulnerable populations may not be covered by Big Data if using sources such as mobile phones are not available to the poorest and most marginalised groups of society.
A vision paper CBS Netherlands and Statistics Canada on future advanced data collection (🔗), presented at the 62nd ISI World Statistics Congress 2019 in Kuala Lumpur, discusses how sensor data and data originating from data platforms (public or private) hosted outside NSOs can play an increased role in the future of data collection and to maximize the benefit of these data sources to produce “smart statistics”.
Smart Statistics can be seen as the future extended role of official statistics in a world impregnated with smart technologies. Smart technologies involve real-time, automated, interactive technologies that optimize appliances and consumer devices’ physical operation. Statistics would then be transformed into a smart technology embedded in smart systems that would transform “data” into “information”.
Some of the main challenges and opportunities outlined in the vision paper are as follows:
Methodology: linking different data sources and validating data that were not collected specifically for official statistical purposes (administrative and sensor data) requires completely new and advanced methodological concepts. Changing from a survey methodology toward a data methodology is key.
Quality: timeliness will become an important characteristic of the quality of statistical products and, of course, timeliness might influence the accuracy of statistical information. Accuracy is not a synonym for quality, but it is one characteristic that determines the quality of statistical products. As long as the accuracy of information is known and specified to the end-user, this will not be a problem. Research into reducing the potential trade-off between timeliness and accuracy would, of course, be of interest.
Data access with respect to social acceptability and legal frameworks: social acceptability is key to gaining access to privately-held data. This means that NSOs need to be transparent and able to demonstrate and explain the value proposition to society (public good) and address society’s concerns about trust, confidentiality and privacy. At the same time, legal frameworks need to be developed to ensure that NSOs can use all these new data sources to their maximum extent and make sure that society can benefit from the added value NSOs can potentially provide.
Data access with respect to technology and methodology: The keywords for future data access are collect, connect and link. Technology to obtain secure data access, in conjunction with the appropriate methodology and algorithms to guarantee privacy and confidentiality, is one of the main technological development areas for the near future. Multi-party computation, privacy-preserving data sharing (PPDS) and privacy-preserving record linkage (PPRL) are potentially promising technological advancements that need to be further developed into a robust set of methods.
Big data in official statistics (🔗), CBS (2020);
Bucharest Memorandum on Official Statistics in a Datafied Society (🔗), 104th DGINS Conference, Bucharest (2018);
Recommendations for access to data from private organizations for official statistics (🔗), Global Working Group on Big Data for Official Statistics (2016);
Scheveningen memorandum on the use of Big Data in official statistics (🔗), Eurostat (2013);
UN Global Working Group on Big Data (🔗).