10.3 Methods and systems of analysis#
10.3.1 Methods of analysis#
Regression and correlation
The most commonly used techniques for investigating the relationship between two quantitative variables are correlation and linear regression.
Correlation quantifies the strength of the linear relationship between a pair of variables, whereas regression expresses the relationship in the form of an equation. Both methods are used in sampling and estimation procedures for sample surveys. They are also used in analysis, particularly to determine the relevance of a research hypothesis. In statistical analysis, correlation can be used to confirm the relation between variables - for example, the turnover of retail trade and the collected value-added tax in the same period are expected to positively correlate.
To determine a regression equation, the first step is to determine the general pattern that the data fits. This includes making a scatter plot and then trying out various equations to find the best fit. It is not always straightforward to select the appropriate regression equation. Experience helps.
Seasonal adjustment and time series
Seasonal adjustment is a method of removing short-time periodic changes based on a basic time series decomposition.
It is widely used in official statistics for removing the seasonal component of a sub-annual (usually monthly or quarterly) time series. In essence, a series is split into four components:
Seasonal component;
Calendar component;
Irregular component; and
Smoothed, seasonally adjusted trend component.
Such decomposition creates a seasonally and calendar adjusted series by the exclusion of the seasonal and calendar components from the original series.
The objective of seasonal adjustment is to facilitate time series analysis, i.e., period to period comparisons in a time series, and detection of the underlying trend, which may otherwise be obscured by seasonal and calendar effects. It involves the removal of seasonal and calendar variations in the original series.
Seasonal adjustment is invariably preceded by pre-treatment, including detection and correction of outliers. The next step is the calendar adjustment, i.e., the removal of trading day variations and moving holiday effects. Then, in some cases, the original series may be differenced, to obtain stationarity, which is a property of a time series required by seasonal adjustment algorithms for them to work properly. The various choices made in setting up a seasonal adjustment (including pre-treatment and calendar adjustment) plan for a particular series are collectively referred to as model selection.
The use of seasonally adjusted time series is becoming the norm in official statistics as users expect the data (particularly short-term data) to be available in a form that is not influenced by seasonal and calendar components. Given that pre-treatment and seasonal adjustment algorithms are complex and computationally intensive, they are invariably implemented using a seasonal adjustment system. There are multiple seasonal adjustment systems available, of which the most commonly used are listed below and further described in Chapter 15.7 โ Specialist statistical processing/analytical software:
New Capabilities and Methods of the X-12-ARIMA Seasonal Adjustment Program (๐).
TRAMO-SEATS, Department of Statistics, National Bank of Spain (๐).
X-13 ARIMA-SEATS system combines X-12 ARIMA and TRAMO-SEATS, developed and supported by the US Census Bureau (๐).
Jdemetra+, also combines X-12 ARIMA and TRAMO-SEATS, developed by the Department of Statistics of the National Bank of Belgium for the ESS Seasonal Adjustment Group (๐).
It is recommended that the seasonal adjustment method, and at least a general procedure for determining the adjustment parameters, are adopted and used consistently within the NSO, and ideally across the entire NSS. Having different seasonal adjustment methods may lead to different seasonally adjusted series of similar initial data sets.
Eurostat - Handbook on Seasonal Adjustment, 2018 edition (๐).
Eurostat - ESS guidelines on temporal disaggregation, benchmarking and reconciliation, 2018 editionย (๐).
ABS - Time Series Analysis: Seasonal Adjustment Method (๐).
Confidentiality rules and disclosure control
Confidentiality is a fundamental principle of statistics discussed in detail in Chapter 3.2.6 โ Principle 6 - Confidentiality. Producers of official statistics must guarantee that individual data collected for statistical compilation, whether they refer to natural or legal persons, are to be strictly confidential and used exclusively for statistical purposes. This section discusses the confidentiality rules that are implemented to ensure the observance of this principle and methods of disclosure control.
The modern approaches to statistical confidentiality distinguishes direct and indirect identification. Direct identification means identification of the respondent from one or a combination of their identifiers (name, address, identification numberโฆ). Indirect identification means inferring a respondentโs identity by combining variables or characteristics such as location combined with age, gender and education. These variables may be found in one data source or in different sources and eventually combined. According to the principle of confidentiality, both direct and indirect identification of a respondent should be avoided. However, access to microdata without identifier but that in some cases could allow indirect identification may be granted for scientific purposes under specific terms and conditions, as further discussed in Chapter 4.5.5 โ User access to confidential data for their own statistical purposes and in Chapter 11.5.3 โ Microdata.
Confidentiality rules for tabular data
Confidentiality rules can be divided into two approaches: active and passive. Passive confidentialising (or confidentialisation) is traditionally limited to international trade in goods statistics, where it is applied only if the dominant enterprise in a tabulation cell (i.e., the enterprise with the largest value) specifically asks for it. Active confidentialising per defined confidentiality rules, is applied in almost all other statistical areas.
NSOs throughout the world most commonly apply the following three confidentiality rules for protecting tabular data:
Number criterion, i.e., applying a minimum requirement of, e.g., three observations in a table cell, for the relevant data in the cell to be published.
Dominance criterion for economic variables (e.g., sales or value-added) is applied. This means that if the largest or two largest businesses together account for a dominant share (e.g., 85%) of the value of a given table cell, confidentialising is applied.
Secondary confidentialising (or residual disclosure); after sensitive cells have been identified and their values suppressed there is still the possibility that the suppressed values can be identified from the values in cells that have not been suppressed. This is referred to as residual disclosure. The simplest example is a one-dimensional table of counts or qualities in which the value of one cell has been suppressed, but the total of all cells is published. In this case, the suppressed cellโs value can readily be deduced by subtracting the values of all other cells from the total. All output tables have to be checked for residual disclosure and other cells suppressed to ensure that it does not occur. The overall number of cells suppressed should be minimised to ensure that as many data as possible are published.
Statistical disclosure control
Statistical disclosure control methods are processes and procedures used to reduce the risk that statistical units are identified when the statistical data are being published. These include:
Tabular data protection for aggregate information on respondents presented in tables (using suppression, rounding and interval publication);
Microdata protection for information on statistical units (using local suppression, sampling, global recoding, top and bottom coding, rounding, rank swapping and micro aggregation).
If the value of a sensitive cell is published, disclosure is said to have occurred, violating the requirement that no confidential data be revealed. Thus, ensuring that there are no sensitive cells in output tables is one requirement for preserving confidentiality. Typically, the value of a sensitive cell is suppressed in the output table, meaning that, instead of being published, it is replaced by an asterisk or other special symbol with a note indicating the reason, i.e., preservation of confidentiality. Automated systems for disclosure control can be integrated into the tabulation solutions, thus providing confidentiality on the fly for any query that the users may request.
Software solutions that automate statistical disclosure control are available on the market, as discussed in Chapter 12.8.5 โ Confidentiality and disclosure control.
10.3.2 Systems for analysis#
Commercial and free open-source systems for data analysis
It is safe to assume that almost every producer of official statistics uses one or more commercial or open-source software packages in the production of statistical data, including for data analysis. While NSOs have used some packages (such as SAS) since the early mainframe days, others such as open-source R have gained popularity more recently.
The purpose of this section is not to recommend the use of a particular software system for analysis, but rather to list possible options and to provide guidance on possible criteria for selection. Below are links to each of the most commonly used statistical software packages. More options are provided in Chapter 15.6 โ Basic IT infrastructure needs and skill requirements and Chapter 15.7 โ Specialist statistical processing/analytical software.
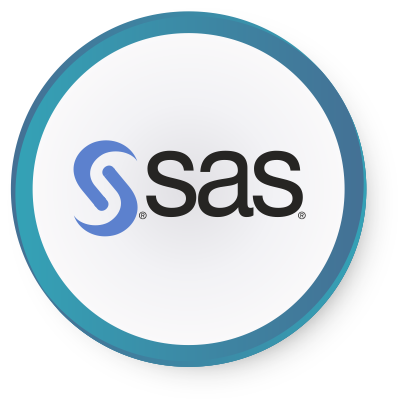
SAS is a software suite that can discover, alter, manage and retrieve data from various sources and perform statistical analysis on them.
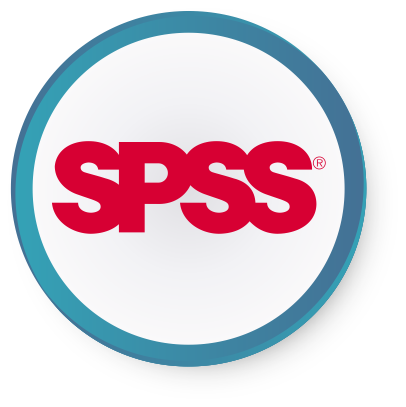
SPSS Statistics is a statistical software platform from IBM by means of which a user can analyse and better understand its data and solve complex business and research problems.
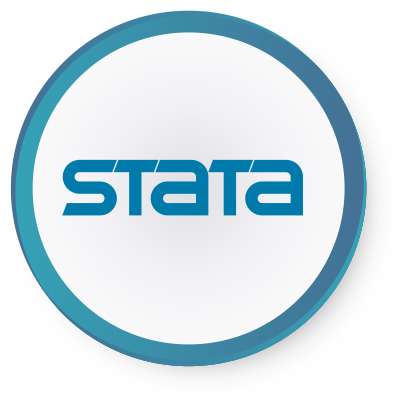
Stata is statistical software that enables users to analyse, manage, and produce graphical visualizations of data.
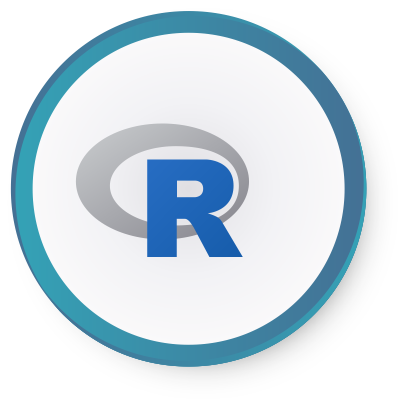
R (Project for Statistical Computing) is a language and environment for statistical computing and graphics.
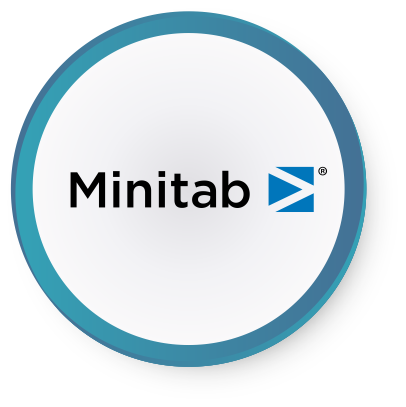
Minitab is a general-purpose statistical software package used as a primary tool for analysing research data.
Selection of the appropriate system for data analysis is often path-dependent. If a particular system is already being used somewhere else in the NSO, it may be difficult and time-consuming to use another system as processes, procedures and customisation are already in place.
Licencing costs are often a limiting factor. Implementing an advanced enterprise-grade statistical system may be too expensive. Availability of local knowledge and training may also nudge an NSO towards a specific solution. Statistical procedures are prewritten in some systems, and recently NSOs have started promoting the sharing of procedures and code, mostly based on open-source platforms (discussed in detail in Chapter 15.2.9 โ Open-source software).
Systems for seasonal adjustment
There are multiple seasonal adjustment packages available of which the most commonly used are listed below and further described in Chapter 15.7 โ Specialist statistical processing/analytical software.
X-12 ARIMA, US Census Bureau (๐).
TRAMO-SEATS, Department of Statistics, National Bank of Spain (๐).
X-13 ARIMA-SEATS system combines X-12 ARIMA and TRAMO-SEATS, developed and supported by the US Census Bureau (๐).
Jdemetra+, also combines X-12 ARIMA and TRAMO-SEATS, developed by the Department of Statistics of the National Bank of Belgium for the ESS Seasonal Adjustment Group (๐).
Systems for confidentiality and disclosure control
As output tables are typically voluminous and may be inter-related, identifying and preventing disclosure is not a process that can readily be done manually. Thus, an NSO should either acquire a confidentiality checking and disclosure control tool or develop a tool of its own. The acquisition is recommended to save development costs. However, as confidentiality checking, and disclosure control tools are not readily available commercially (there being very little demand for them outside the realm of official statistics) acquisition is likely to be from another NSO. Two well-known examples are as follows and further described in Chapter 12.8.5 โ Confidentiality and disclosure control:
ARGUS, Statistics Netherlands: as described in ARGUS Usersโ Manual Version 3.3 (๐), the purpose of ฮ-ARGUS is to protect tables against the risk of disclosure. This is achieved by modifying the tables so that they contain less detailed information. A twin application, ยต-ARGUS protects microdata files. Both applications have been rewritten in open source (๐).
G-Confid, Statistics Canada: as described in GโConfid: Turning the tables on disclosure risk, 20 (๐), G-Confid is a generalized system that can deal with potentially voluminous multi-dimensional tables and that can incorporate new approaches.